AI and ML have evolved from futuristic concepts to powerful technologies that are transforming how users and businesses make decisions. In the big data sector, AI and ML are crucial for extracting valuable, in-depth insights from raw data, enabling timely and precise decision-making. Snowflake is at the core of the AI revolution, attempting to make AI more accessible, scalable, and secure for data-driven enterprises. Their most recent releases and breakthroughs in 2024 have the potential to fundamentally change how people interact with data, thereby strengthening AI as an essential tool for data experts.
In this article, we will explore the core updates and releases from Snowflake AI announced in 2024, highlighting their importance and applications—and much more!
Let's dive right in!
Core Snowflake AI Updates and Releases (2024)
This year, 2024, has been a fully exciting year—we've seen many awesome AI products and features. Snowflake also released a bunch of improvements to their AI product lineup, designed to benefit both users and enterprises. In this section, we will explore Snowflake's most significant releases and advancements.
1) Snowflake Arctic
Snowflake Arctic, a state-of-the-art large language model developed by Snowflake's AI research team, was unveiled on April 24, 2024. Snowflake Arctic is a groundbreaking achievement in enterprise AI, combining superior intelligence with exceptional efficiency and a strong commitment to transparency. Snowflake Arctic utilizes a unique Dense Mixture of Experts (MoE) Hybrid transformer architecture, with 480B parameters distributed among 128 fine-grained experts, and top-2 gating to choose 17B active parameters.
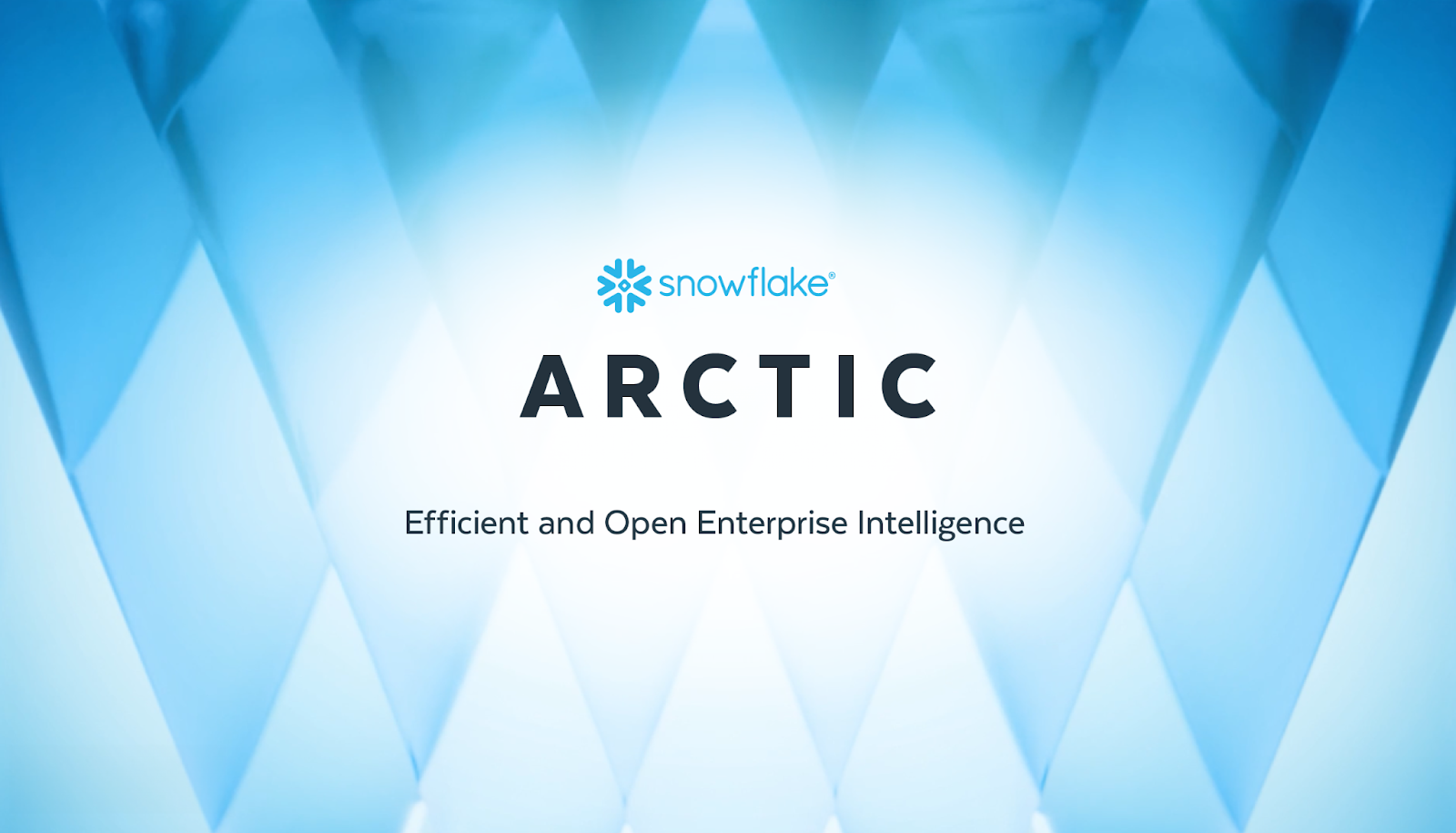
Here are some key features that distinguish it from other LLMs in the industry:
1) Intelligent—Snowflake Arctic excels at complex enterprise tasks such as SQL generation, coding, and instruction following.
2) Highly Efficient—Snowflake Arctic is powered by a Dense-MoE Hybrid transformer architecture, it delivers top-tier performance at a fraction of the development cost compared to similar models.
3) Openness—Snowflake Arctic is released under the Apache 2.0 license, it provides open access to its weights and code.
4) Enterprise AI Focused—Snowflake Arctic is specifically tailored for enterprise AI needs, it focuses on high-quality tasks like data analysis and automation. It excels in core applications and outperforms larger models without requiring extensive computing resources, making it a cost-effective solution for enterprises.
Snowflake Arctic Models
Snowflake Arctic Instruct is fine-tuned to generate high-quality responses from natural language prompts, making it ideal for conversational AI applications within enterprises.
Snowflake Arctic Base is a pre-trained foundational model that can be used in various applications without further fine-tuning. It is versatile and ready to use, suitable for tasks such as retrieval-augmented generation (RAG)
Snowflake also introduced the Snowflake Arctic family of text embedding models in mid-April 2024, which includes five text embedding models tailored for retrieval tasks and licensed under the Apache 2.0 license. The models are:
- Snowflake-arctic-embed-m
- Snowflake-arctic-embed-l
- Snowflake-arctic-embed-m-long
- Snowflake-arctic-embed-xs
- Snowflake-arctic-embed-s
For a more in-depth and comprehensive guide on Snowflake Arctic, watch this video.
Or
Check out our detailed coverage on Snowflake Arctic.
Want to take Chaos Genius for a spin?
It takes less than 5 minutes.
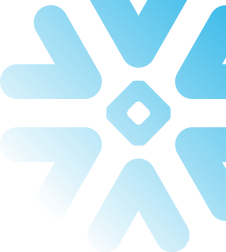
2) Snowflake Cortex
Snowflake Cortex is a fully managed service that integrates advanced AI and ML solutions directly within the Snowflake environment, enabling users to leverage their data—structured or unstructured—effectively. Snowflake Coretx can help you to fully dive deep into your data, seamlessly analyze it, and build powerful AI applications with pre-built, industry-leading models and vector search capabilities, all within the Snowflake platform.
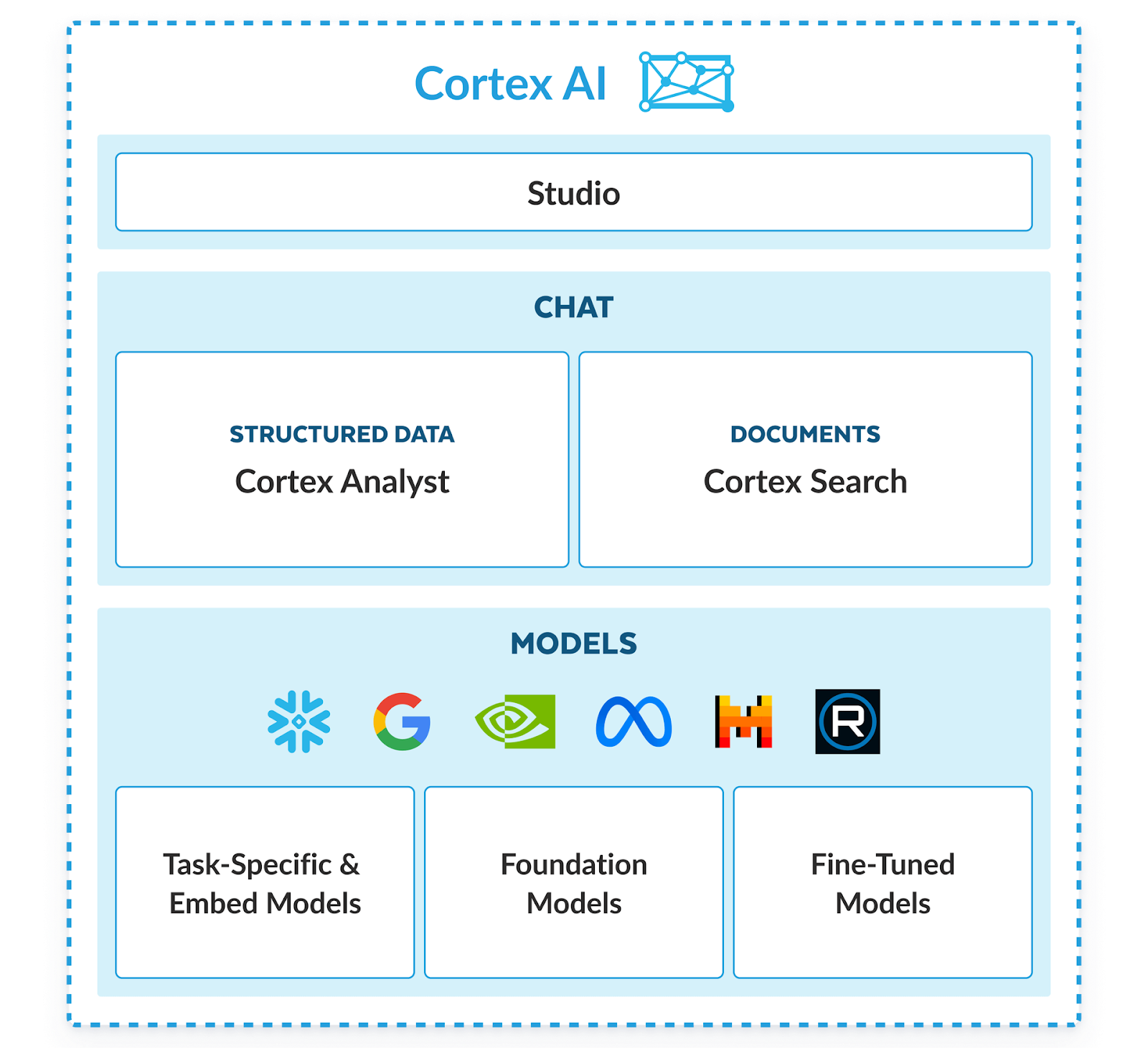
Here are the key features and benefits of Snowflake Cortex:
1) Seamless Integration—Snowflake Cortex integrates smoothly with the Snowflake, reducing the need for complex setups or data transfers between systems, allowing users to instantly use AI and ML capabilities without additional overhead.
2) High Performance—Snowflake Cortex uses Snowflake's scalable architecture to efficiently manage AI and ML workloads, allowing end users to do complex data analysis and build AI applications without performance bottlenecks.
3) Top-notch Security and Governance—All data processed within Snowflake Cortex is kept within Snowflake's secure perimeter, benefiting from the platform's strong security features and governance controls. Role-based access control and data privacy safeguards are used to guarantee compliance and security.
4) Cost-Effective—Snowflake Cortex uses a pay-per-use pricing model, making advanced AI and ML capabilities more inexpensive and accessible. Users simply pay for what they consume.
5) Rapid Insights—Snowflake Cortex accelerates data insights, simplifying AI and ML infrastructure development and management. This simplifies AI and ML implementation, allowing users to focus on actionable insights.
Snowflake Cortex LLM Functions
Snowflake Cortex LLM functions provide instant access to industry-leading large language models (LLMs) such as mistral-large, mixtral-8x7b, llama2-70b-chat, and gemma-7b. These models enable various natural language processing (NLP) tasks like text generation, classification, and translation all within Snowflake.
Here are all the supported Snowflake Cortex LLM functions and their key capabilities:
1) COMPLETE
This function generates text based on a provided prompt. It's useful for tasks such as content generation, chatbot responses, and code generation.
This function is designed to extract specific information from unstructured data based on a query, it is perfect for retrieving insights from documents, manuals, and large datasets. It simplifies finding relevant information without extensive data manipulation.
3) SENTIMENT
This function analyzes the sentiment of a given text, providing a score that indicates whether the sentiment is positive, negative, or neutral. It's particularly useful for understanding customer feedback, social media monitoring, and other applications where gauging emotional tone is crucial.
4) SUMMARIZE
This function generates concise summaries of longer texts, making it easier to digest large volumes of information. It's beneficial for summarizing product reviews, and any long-form content that needs to be quickly understood.
5) TRANSLATE
This function translates text between languages, supporting multilingual capabilities for applications that require real-time translation services.
Check out our in-depth coverage of Snowflake Cortex LLM function.
Snowflake ML Functions
Snowflake Cortex ML functions offer powerful tools for machine learning tasks directly within the Snowflake environment, allowing data analysts and engineers to create and use machine learning models without needing extensive ML expertise.
The available Snowflake Cortex ML functions can be broadly categorized into two groups:
- Forecasting: This function predicts future values based on historical time-series data. It is particularly useful for tasks like sales forecasting, demand prediction, and other scenarios where understanding future trends based on past data is crucial. The forecasting function can handle exogenous variables (external factors) to improve prediction accuracy.
- Anomaly Detection: This function identifies outliers or anomalies in your data, which are data points that deviate significantly from the norm. It is useful for fraud detection, quality control, and monitoring any unexpected deviations in metrics over time.
- Contribution Explorer: This function helps identify which factors or dimensions significantly impact a given metric. It's useful for understanding the underlying causes of changes in key business metrics, such as sales or user engagement.
- Classification: The classification function sorts data into predefined classes using patterns identified in the training data. It supports both binary and multi-class classification. Use cases include customer segmentation, churn prediction, and categorizing transactions or behaviors based on historical data.
For more detailed information and practical examples, See Snowflake Documentation on ML Functions.
3) Universal Search
Snowflake Universal Search is a powerful search functionality within the Snowflake platform. Built on top of Snowflake Cortex, Universal Search allows users to quickly find a wide array of objects and resources across their accounts. It enables users to search for database objects, data products in the Snowflake Marketplace, relevant Snowflake documentation, and community knowledge base articles using natural language queries.
Universal Search is designed to understand and interpret user queries, even accommodating misspellings or partial terms, making it easier to locate relevant data and resources.
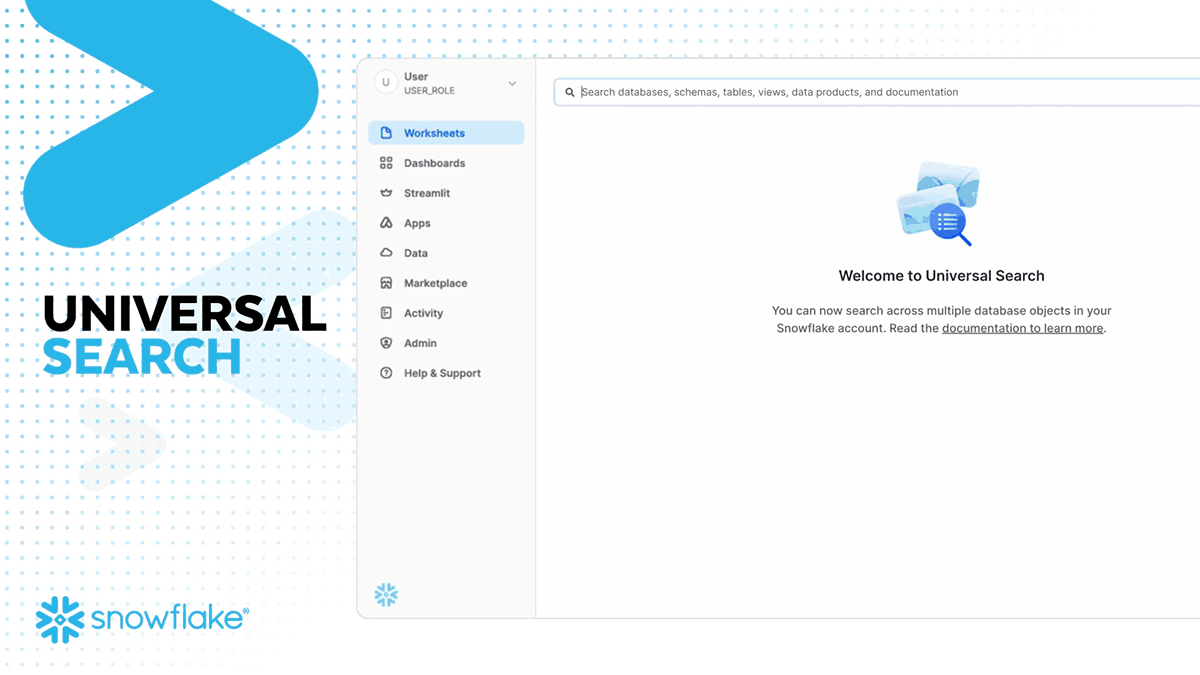
Step-by-Step Guide to Using Snowflake Universal Search in Snowflake Snowsight
Step 1—Sign in to Snowflake Snowsight
Open your web browser and navigate to the Snowflake Snowsight login page and then enter your credentials to sign in to your Snowflake account.
Step 2—Access the Search Feature
Once logged in, locate the navigation menu on the left side of the screen. Click on the "Search" option to open the Universal Search interface.
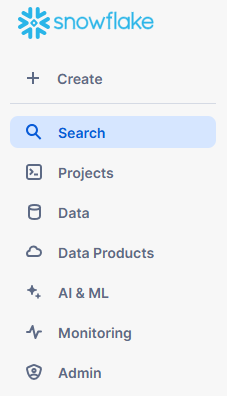
Step 3—Enter Search Terms
In the search bar, type in your search terms using keywords or natural language. Press the Return key to initiate the search.
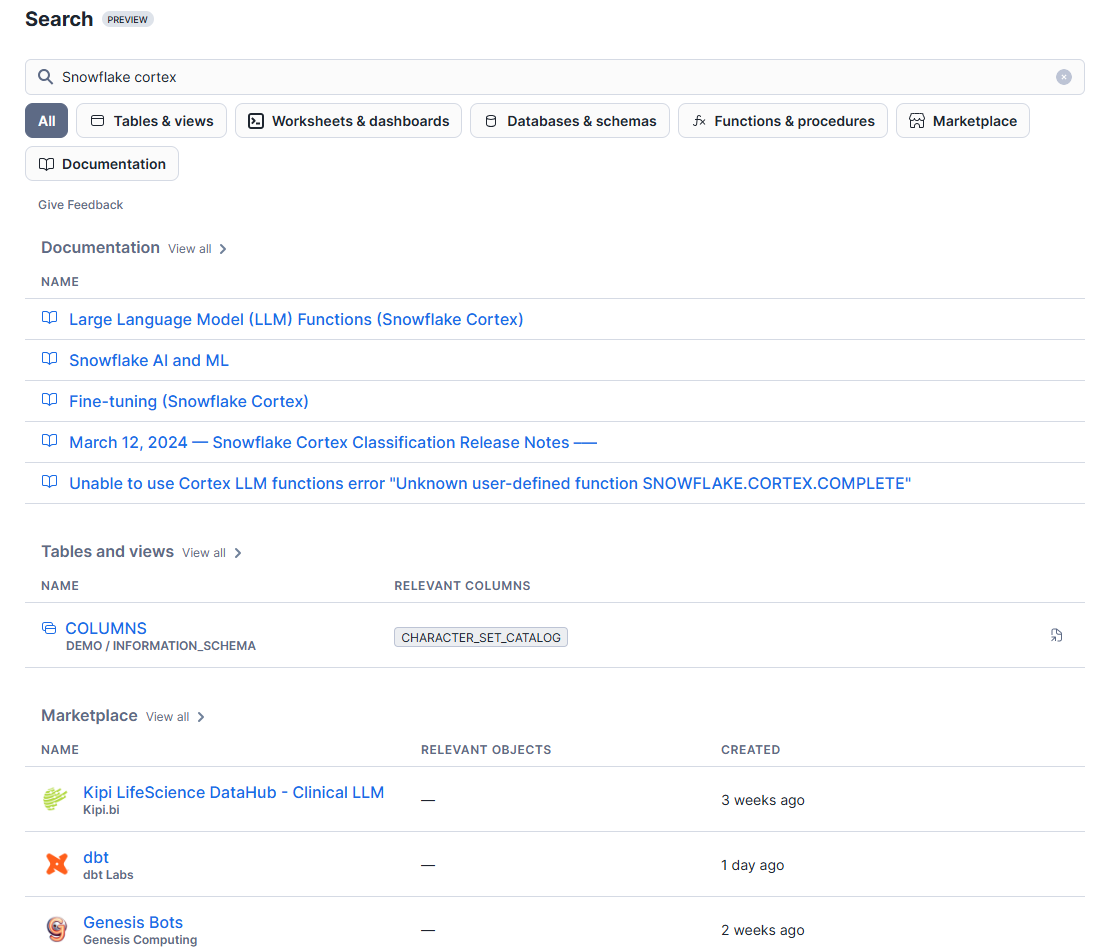
Step 4—Review Search Results
The search results will be displayed in categorized sections, such as Tables, Views, Documentation, Data Products, etc. If a category isn’t displayed, it means there are no results for that category or your currently active role does not have access to those results.

Step 5—Select a Search Result
Click on a search result to view more details. For database objects like tables or views, you can select the "Open in Worksheets" option to query the object directly in a worksheet.
Step 6—Access Marketplace Listings
If your search returns listings from the Snowflake Marketplace, you can get or purchase these listings. Make sure you have agreed to the Snowflake Provider and Consumer Terms to access these listings.
Limitations of Snowflake Universal Search
1) Supported Object Types—Snowflake Universal Search only returns results for dashboards, worksheets, databases, schemas, tables, views, user-defined functions (UDFs), stored procedures, data products in the Snowflake Marketplace, documentation pages, and knowledge base articles.
2) Indexing Delay—New objects may take a few hours to appear in search results after creation. Similarly, objects that are dropped and recreated may temporarily disappear from search results until re-indexed.
3) Role-Based Access—Snowflake Universal Search results are filtered based on the privileges of the currently active role. You will only see results that your active role has permission to view.
4) Non-Searchable Content—Snowflake Universal Search only indexes object metadata (names, comments, tags) and does not search the actual contents of database objects.
5) Availability—Snowflake Universal Search is not yet available in Virtual Private Snowflake (VPS) or government cloud regions.
6) Language Support—The search functionality is optimized for English search terms.
4) Snowflake Copilot
Snowflake Copilot is an LLM-powered assistant designed to simplify data analysis within the Snowflake ecosystem. It integrates seamlessly into the Snowflake workflow, guaranteeing robust data governance and security. Powered by a model fine-tuned by Snowflake and running inside Snowflake Cortex, Snowflake Copilot processes natural language requests to assist users in data exploration, SQL query generation, and understanding Snowflake features.
Snowflake Copilot is currently available in the following regions:
- AWS us-east-1
- AWS us-west-2
- AWS EU-central-1
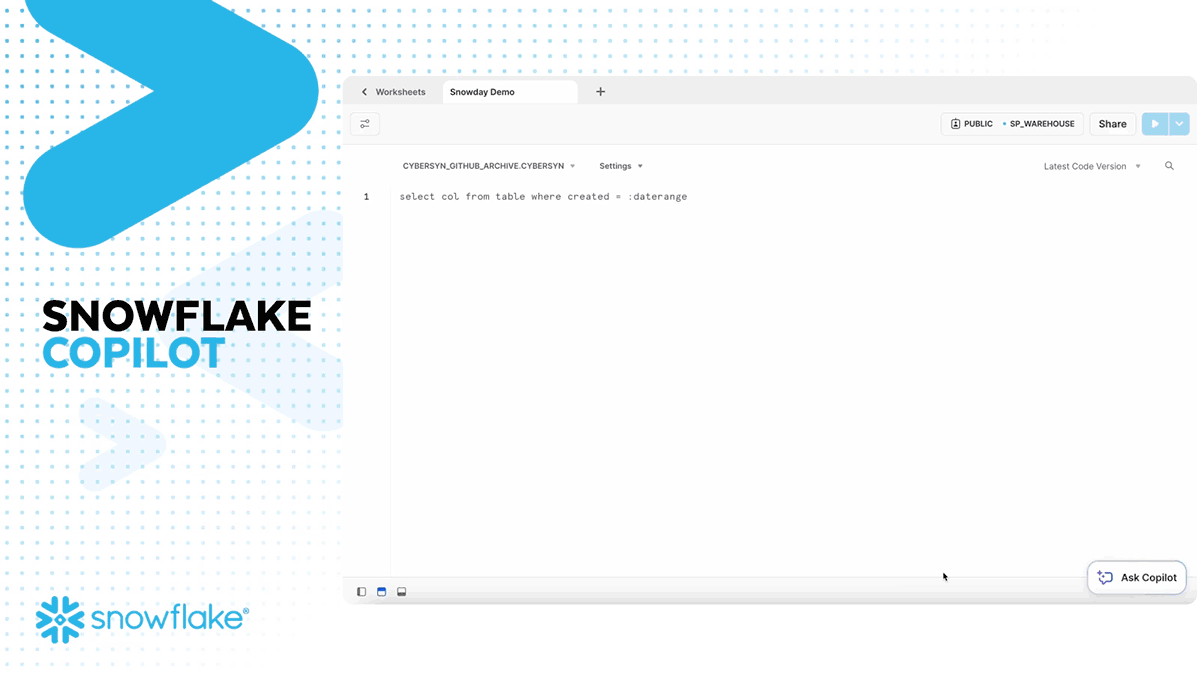
Use Cases of Snowflake Copilot
Here are some of the use cases of Snowflake Copilot:
- Data Exploration—Ask open-ended questions to learn about new datasets.
- SQL Query Generation—Create SQL queries using plain English prompts.
- SQL Query Execution—Run or edit suggested SQL queries directly.
- Complex Query Building—Refine SQL queries through iterative conversations.
- Learning Snowflake—Inquire about Snowflake concepts, capabilities, and features.
- Query Optimization—Request improvements or explanations for SQL queries.
- Feedback Integration—Provide feedback to improve Copilot's responses.
- Custom Instructions—Add specific business knowledge or preferences for personalized responses.
Limitations of Snowflake Copilot
Here are some of the limitations of Snowflake Copilot:
- Snowflake Copilot only supports English and SQL.
- Snowflake Copilot cannot access the actual data inside tables; users must provide specific values for filters.
- Snowflake Copilot does not support queries across different databases or schemas.
- Snowflake Copilot responses may take a few seconds, especially for longer queries.
- Snowflake Copilot may occasionally suggest queries with invalid syntax or non-existent tables/columns.
- Snowflake Copilot may take up to 3-4 hours to detect new databases, schemas, or tables.
- Snowflake Copilot considers only the top 10 relevant tables and columns for responses.
5) Snowflake Document AI
Snowflake Document AI is a Snowflake AI feature that uses a proprietary large language model called Arctic-TILT to extract data from various types of documents. It can handle text-heavy content as well as graphical elements like logos/images, handwritten text (such as signatures), and checkmarks. Snowflake Document AI also allows you to create pipelines for continuous processing of specific types of documents, such as invoices or financial statements.
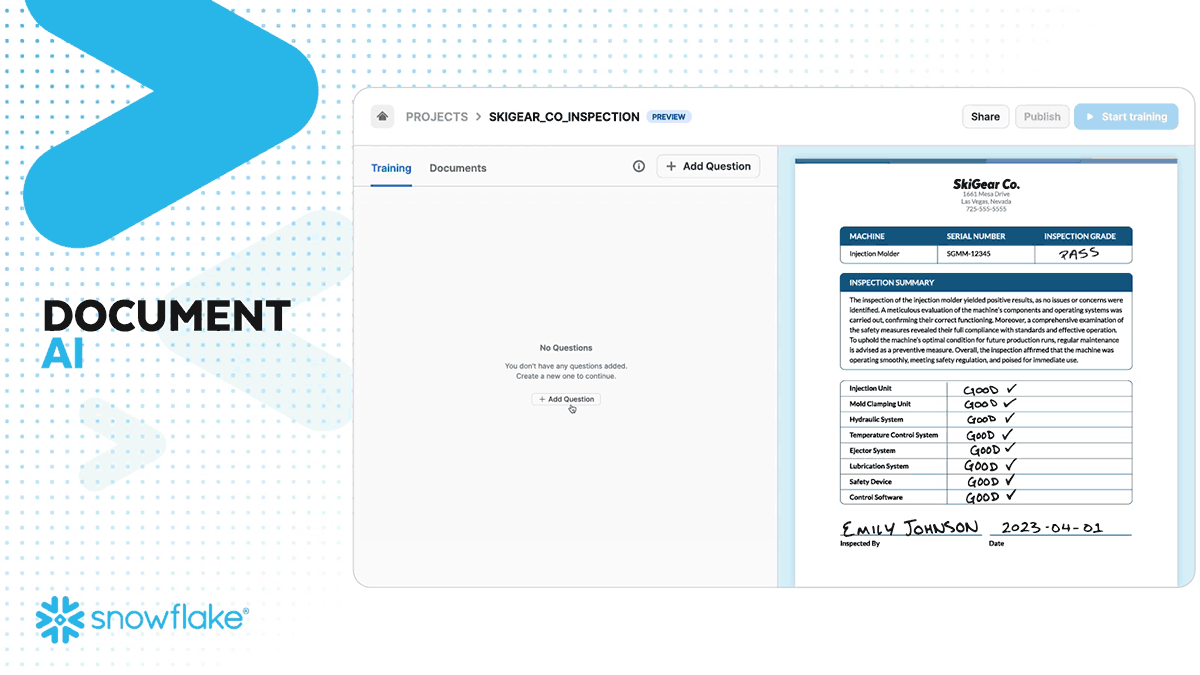
Document AI should be used only when you need to convert unstructured data from documents into structured data in tables. It is also ideal for setting up automated pipelines to continuously process new documents of a specific type.
Also, Document AI is beneficial when business users with domain knowledge are involved in preparing the model, and data engineers work on setting up the pipelines to automate the processing.
See Document AI in action:
6) Cortex Fine-tuning
Fine-tuning with Snowflake Cortex lets you customize LLMs for specific tasks without the high costs of training from scratch. This feature uses parameter-efficient fine-tuning (PEFT) to adapt pre-trained models for specialized tasks, improving results beyond what prompt engineering or retrieval augmented generation (RAG) methods can achieve.
Fine-tuning allows users to adjust the behavior of an existing large language model by providing examples of the desired input and output pairs. This process trains the model on the user's data, improving its knowledge and performance for domain-specific tasks.
Snowflake Cortex Fine-Tuning lets users fine-tune popular large language models using their data, all within the Snowflake environment. Snowflake Cortex Fine-Tuning features are available through the Snowflake Cortex function, FINETUNE, with these arguments:
- CREATE: Initiates a fine-tuning job using the specified training data.
- SHOW: Lists all fine-tuning jobs accessible by the current role.
- DESCRIBE: Provides the progress and status of a specific fine-tuning job.
- CANCEL: Terminates a specific fine-tuning job.
Limitations and Considerations of Snowflake Cortex Fine-Tuning
Here are a few key limitations of fine-tuning Snowflake Cortex:
1) Additional costs—On top of the charges for tuning and inference, users will incur regular storage and warehouse costs for storing the customized adaptors and running SQL commands.
2) Long-running jobs—Fine-tuning jobs are often long-running and are not attached to a worksheet session.
3) Model availability—If a base model is removed from the Snowflake Cortex LLM Functions, the fine-tuned model created from it will no longer work.
4) Access control requirements—To run a fine-tuning job, the role that creates the job needs specific privileges, such as USAGE on the database containing the training data and CREATE MODEL or OWNERSHIP on the schema where the model is saved.
5) Limited models—Only a few pre-trained base models are currently available for fine-tuning, such as:
6) Training data requirements—The training data must be in a Snowflake table or view with columns named "prompt" and "completion". The input context (prompt) and output context (completion) have specific token limits based on the base model being fine-tuned.
7) Visibility and permanence—Fine-tuning jobs are listable at the account level only, and the job listings returned from the FINETUNE ('SHOW') function may be periodically garbage collected.
Step-by-Step Guide to Fine-Tune Models Using Snowflake Cortex
Here is the step-by-step guide to fine-tuning Models using Snowflake Cortex.
Step 1—Prepare the Training Data
Training data must come from a Snowflake table or view with columns named prompt and completion. If your table or view does not contain columns with the required names, use a column alias in your query to name them appropriately.
Note: All columns other than prompt and completion will be ignored by the FINETUNE function. Snowflake recommends selecting only the necessary columns.
Step 2—Additional Recommendations for Training Data
Start with a few hundred examples to avoid drastic increases in tuning time with minimal performance improvements. Make sure that the examples fit within the allotted context window for the base model.
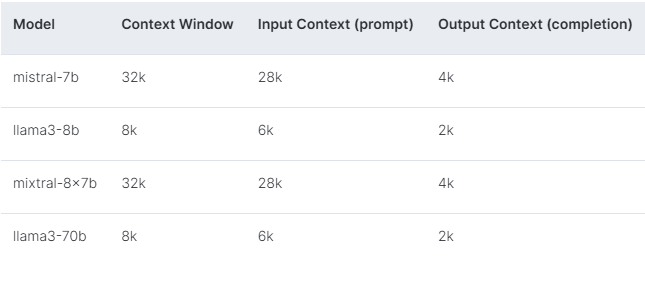
Step 3—Start the Fine-Tuning Function
Use the SNOWFLAKE.CORTEX.FINETUNE function with CREATE as the first argument to start the job.
USE DATABASE mydb;
USE SCHEMA myschema;
SELECT SNOWFLAKE.CORTEX.FINETUNE(
'CREATE',
'my_tuned_model',
'mistral-7b',
'SELECT prompt, completion FROM my_training_data',
'SELECT prompt, completion FROM my_validation_data'
);
Step 4—Monitor the Training Job
Use the SNOWFLAKE.CORTEX.FINETUNE function with DESCRIBE as the first argument to get the status or job progress.
SELECT SNOWFLAKE.CORTEX.FINETUNE(
'DESCRIBE',
'your_job_id'
);
Step 5—Manage Fine-Tuning Jobs
If you need to terminate a fine-tuning job, use the CANCEL argument.
SELECT SNOWFLAKE.CORTEX.FINETUNE(
'CANCEL',
'your_job_id'
);
Step 6—Use Your Fine-Tuned Model for Inference
Use the Snowflake Cortex COMPLETE LLM function with the name of your fine-tuned model.
SELECT SNOWFLAKE.CORTEX.COMPLETE(
'my_tuned_model',
'<your prompt>'
);
See LLM Fine-tuning in action with Snowflake Cortex AI.
7) Snowpark ML
Snowpark ML is an integrated framework within Snowflake designed for end-to-end machine learning. It enables data scientists and ML engineers to develop, train, and deploy ML models directly on Snowflake's platform, utilizing its powerful data management and governance features. Snowpark ML supports both custom and out-of-the-box workflows, making it easy to manage the entire ML lifecycle securely and efficiently.
For more detailed information, see Snowpark ML documentation.
These are the core tools Snowflake has added to their platform, but they have introduced even more in their AI product lineup. They've released numerous impressive add-ons, functions, and features, which we will cover in the next section.
More Enhanced Snowflake AI Capabilities—and Integrations
Snowflake has also launched several new AI features and integration capabilities to enhance its platform. These developments are designed to deliver deeper insights, more efficient data processing, and smoother connections with other tools and services, allowing users and enterprises to better use AI in their operations.
❄️ EMBED_TEXT Functions
Snowflake EMBED_TEXT functions enable users to create vector embeddings for text input, which is useful in sophisticated applications such as semantic search, clustering, and similarity analysis. These embeddings convert textual data into numerical vectors, making it easier to perform complex operations on large datasets.
Snowflake currently offers three primary EMBED_TEXT functions, each leveraging different underlying models to suit various use cases:
1) EMBED_TEXT_768 (snowflake-arctic-embed-m)
This function creates 768-dimensional embeddings using the snowflake-arctic-embed-m model. It is optimized for medium-sized tasks and offers efficient performance with a manageable number of parameters, making it suitable for most enterprise applications.
2) EMBED_TEXT_768 (e5-base-v2)
Similar to the first, this function also generates 768-dimensional embeddings but uses the e5-base-v2 model. This model is designed for general-purpose text embedding tasks, providing a balance between performance and computational cost.
3) EMBED_TEXT_1024 (nv-embed-qa-4)
This function produces 1024-dimensional embeddings using the nv-embed-qa-4 model. It is tailored for high-precision tasks, such as detailed semantic analysis and complex search operations, where higher dimensionality can offer better accuracy.
❄️ VECTOR data type | Vector Similarity Functions | Vector Embedding Function
Vector Data Type
VECTOR data type in Snowflake is designed to efficiently encode and process vector data, supporting applications that require semantic vector search and retrieval. This data type is crucial for applications such as Retrieval-Augmented Generation (RAG) and other vector-processing tasks.
Vectors are defined in Snowflake with the syntax VECTOR(<type>, <dimension>), where type can be either 32-bit integers (INT) or 32-bit floating-point numbers (FLOAT), and dimension specifies the length of the vector, capped at 4096. This structure allows for efficient handling of high-dimensional data, crucial for tasks involving large-scale vector operations.
Vector Similarity Functions
Vector similarity functions measure the similarity between vectors—a fundamental operation in semantic comparison, enabling applications such as semantic search and enhancing AI responses by providing contextually relevant documents.
Snowflake Cortex provides three main vector similarity functions:
- Computes the inner product (dot product) of two vectors.
- Useful for determining the similarity of vector magnitudes and directions.
- Measures the Euclidean (L2) distance between two vectors.
- Perfect for applications requiring direct distance metrics in multi-dimensional space.
- Computes the cosine similarity between two vectors, reflecting the angular distance.
- Effective for comparing orientation in a multi-dimensional space, independent of magnitude.
Vector Embedding Function
Vector embeddings transform high-dimensional data, such as text or images, into a structured numerical representation—vectors—preserving semantic similarities. This dimensionality reduction is achieved through advanced deep learning techniques, enabling efficient and meaningful comparisons of complex data.
Snowflake Cortex offers vector embedding functions like EMBED_TEXT_768 and EMBED_TEXT_1024. These functions convert text into vector embeddings, facilitating semantic comparisons and enhancing search capabilities beyond simple keyword matching.
For Example:
SELECT SNOWFLAKE.CORTEX.EMBED_TEXT_768('snowflake-arctic-embed-m', 'sample text');
❄️ Enhancements to Snowflake Data Clean Rooms
Snowflake Data Clean Rooms provide a secure and privacy-preserving environment where multiple parties can collaborate and analyze data without revealing their raw data. These clean rooms enable data providers to share insights with data consumers while controlling access and guaranteeing privacy through various techniques such as differential privacy and encrypted multi-party computations.
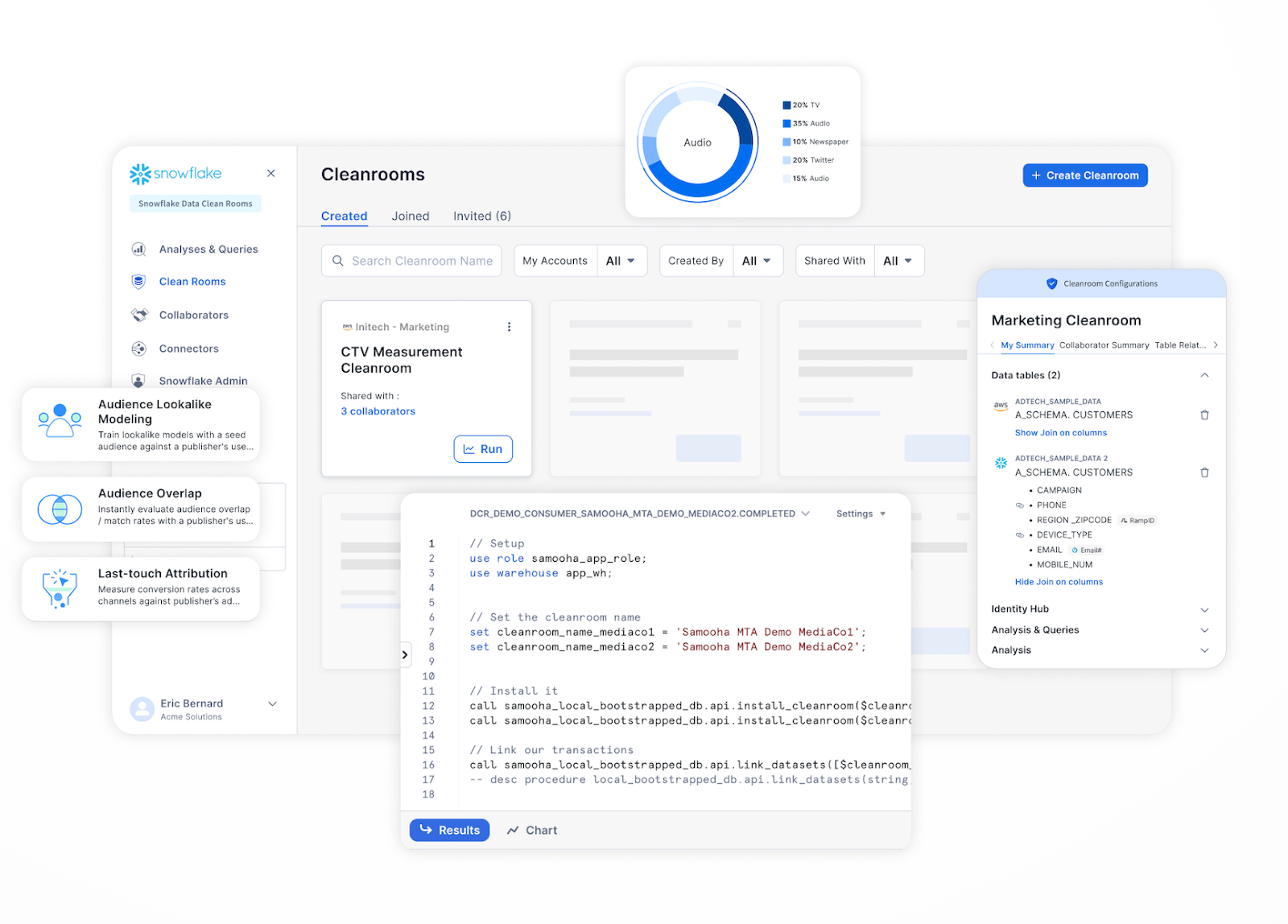
Here are some of the key features provided by Snowflake Data Clean Rooms:
1) Fully Privacy-Focused Collaboration—Snowflake Data Clean Rooms use techniques such as differential privacy and encrypted multi-party computation to ensure data privacy while enabling secure collaboration on sensitive or regulated data.
2) Controlled Data Sharing—Providers can define what analyzes consumers can perform within the Snowflake Data Clean Room, preventing direct access to raw data and maintaining strict data governance.
3) Flexible Collaboration—Snowflake Data Clean Rooms support scenarios where collaborators can act as both providers and consumers. This flexibility extends even to non-Snowflake users through managed accounts, making it easier for diverse parties to collaborate securely.
4) User-Friendly Interfaces—Snowflake Data Clean Rooms offer both a web app for business users and developer APIs for technical users, enabling a wide range of customization and programmatic interactions within the clean rooms.
Latest Enhancements on Snowflake Data Clean Rooms
In the latest update, Snowflake introduced several enhancements to Data Clean Rooms, making them more versatile and user-friendly. Here are the updates:
1) Multi-provider Clean Rooms (General Availability):
One major update is the availability of multi-provider clean rooms through the developer APIs. This allows consumers to run analyses across multiple clean rooms, combining data from different providers while maintaining each provider's security controls.
2) Additional Supported Regions (General Availability):
Snowflake also expanded the availability of Data Clean Rooms to more regions, including new areas on Google Cloud Platform and various locations in Europe. The following new regions are supported:
North America:
- Google Cloud Platform: US Central1 (Iowa), US East4 (N. Virginia)
Europe:
- Amazon Web Services: EU (Ireland), Europe (London), EU (Paris), EU (Frankfurt), EU (Stockholm)
- Microsoft Azure: UK South (London), North Europe (Ireland), West Europe (Netherlands), Switzerland North (Zurich)
- Google Cloud Platform: Europe West2 (London), Europe West4 (Netherlands)
3) Support for Views in the Web App (General Availability):
The web app now supports the use of views, materialized views, and secure views within clean rooms. This means that users can include these types of views in their analyses, provided that all necessary usage permissions are granted.
4) Clean Room Customizations for Identity & Activation (General Availability):
Customizations for identity and activation have been improved as well. Providers can now tailor which partners are available for data activation within a clean room, ensuring that consumers use only the preferred options set by the provider. This customization is managed through a new section in the admin settings (Admin » Clean Room Features menu).
5) Custom Template Enhancements (General Availability):
Lastly, enhancements to custom templates make them more flexible. Developers can now add date selectors to the user interfaces of their templates and hide default table selectors if needed. These improvements are accessible via the provider API, allowing for a more customized and streamlined user experience.
❄️ Snowflake Native Apps with Snowpark Container Services
Snowflake Native App Framework allows developers to create and distribute data applications within the Snowflake ecosystem. These applications leverage Snowflake's core functionality and enable seamless data sharing and business logic execution across different Snowflake accounts. Key components of the framework include:
1) Providers and Consumers—Providers create and share applications, while consumers install and use them.
2) Application Packages—These encapsulate data content, application logic, metadata, and setup scripts.
3) Streamlined Development and Deployment—The framework offers a robust developer workflow, versioning, and patching capabilities, and supports structured and unstructured event logging for monitoring and troubleshooting.
4) Snowflake Marketplace and Private Listings—Applications can be distributed and monetized via the Snowflake Marketplace or through private listings to specific consumers.
5) Integration with Streamlit—Applications can include rich visualizations created with Streamlit.
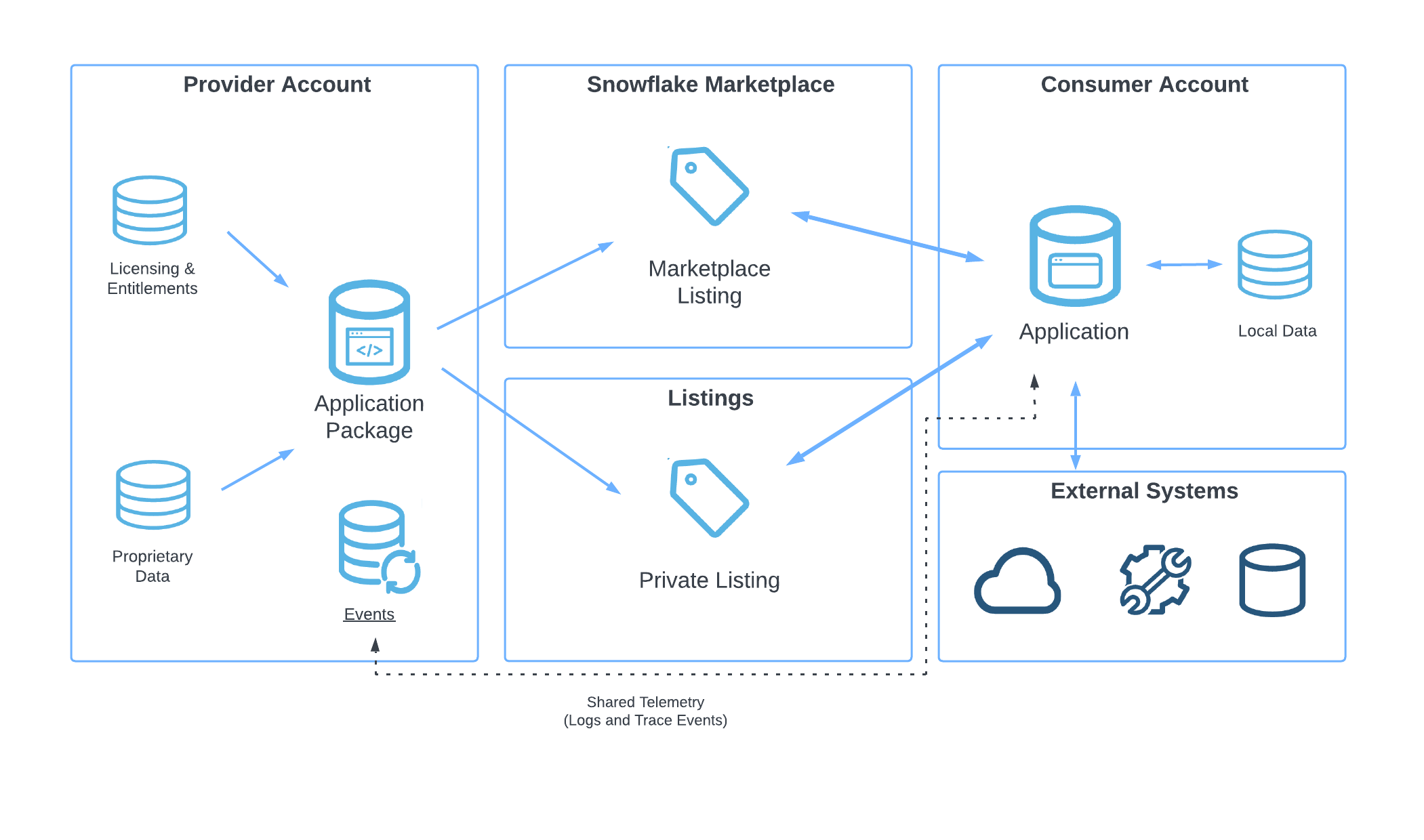
For a more indepth guide, check out Snowflake Native Apps with Snowpark Container Services.
Latest Enhancements with Snowflake Native Apps and Snowpark Container Services
Recent updates to the Snowflake Native Apps framework include the integration of Snowpark Container Services, enhancing the capabilities of Snowflake Native Apps.
These enhancements are as follows:
1) Support for Containerized Services
Snowflake Native Apps can now run any containerized service supported by Snowpark Container Services within the Snowflake environment. This allows for more complex and flexible application workloads, leveraging containerized environments.
2) Enhanced Application Package
The application package for containerized apps includes a services specification file that references the required container images. These images must be stored in an image repository in the provider’s account.
3) Application Object with Compute Pool
When installed, the application object for containerized apps includes a compute pool, which is a collection of virtual machine nodes that run the Snowpark Container Services jobs and services. Users can either grant the CREATE COMPUTE POOL privilege to the app or manually create compute pools.
4) Provider IP Protection
To protect provider intellectual property, the framework redacts sensitive information from the QUERY_HISTORY and ACCESS_HISTORY views. The query profile graph is simplified to prevent exposure of detailed execution plans.
Latest Collaborations and Partnerships
Snowflake and NVIDIA Partnership
In June 2024, Snowflake announced a groundbreaking collaboration with NVIDIA to enable the development of customized AI applications within Snowflake, leveraging NVIDIA's AI technology. This partnership integrates NVIDIA's accelerated computing and software with Snowflake's robust data infrastructure, particularly within Snowflake Cortex AI.
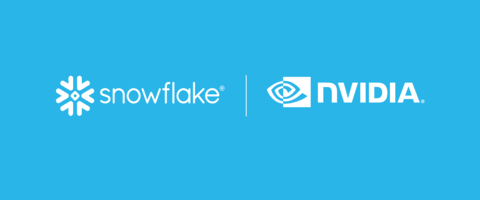
This collaboration allows Snowflake to offer a full-stack AI platform that combines NVIDIA's accelerated computing with Snowflake's robust data infrastructure.
Key highlights of this partnership:
Integration of NVIDIA AI Enterprise Software—Snowflake has integrated NVIDIA AI Enterprise software to enhance its Cortex AI platform. This integration includes technologies like NeMo Retriever, which facilitates accurate custom model deployment.
Optimization with NVIDIA TensorRT-LLM—Snowflake Arctic LLM has been optimized with NVIDIA TensorRT-LLM for high performance, making it available as an NVIDIA NIM inference microservice.
Full-Stack AI Platform—The collaboration brings together NVIDIA's computing power and Snowflake's data infrastructure, enabling users to develop AI applications rapidly and efficiently. This includes the use of NVIDIA Triton Inference Server and other AI capabilities within Cortex AI, allowing for the creation of sophisticated AI solutions tailored to specific use cases
Because of this partnership, Snowflake users can now quickly create custom AI solutions tailored to their specific needs, using their own data.
Snowflake and Mistral AI Partnership
In March 2024, Snowflake partnered with Mistral AI to bring Mistral's leading language models to the Snowflake Data Cloud. This partnership includes an investment from Snowflake Ventures in Mistral AI's Series A funding, emphasizing their commitment to advancing AI capabilities.
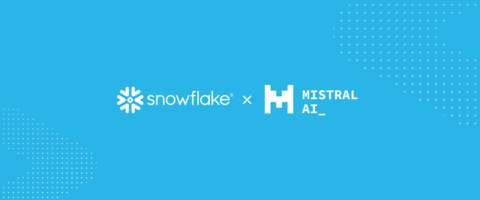
Key highlights of this partnership:
Access to High-Performance Language Models—Snowflake users can utilize Mistral AI's flagship language models, including 'Mistral Large', Mixtral 8x7B, and Mistral 7B, directly within the platform through Snowflake Cortex.
Multilingual Proficiency and Diverse Applications—Mistral AI's models excel in multiple languages and are proficient in tasks such as code generation and mathematical reasoning, expanding the scope of AI applications within Snowflake.
Because of this partnership, everyone can now easily and securely build and deploy AI solutions without leaving the platform.
Apart from the Snowflake AI releases, Snowflake also introduced and announced several core updates to their platform at the Snowflake Data Cloud Summit. Let's dive into what they announced this year.
Snowflake Data Cloud Summit 2024 — Announcements and Releases
Several new features and enhancements were unveiled at the Snowflake Data Cloud Summit 2024, some of which we've already highlighted in the core Snowflake updates above. Here are the significant updates from the event:
1) Snowflake Snowsight updates
Snowflake Snowsight now includes multiple appearance modes (themes) to enhance user experience. The available modes are:
- Light Mode: Displays dark text on a light background, ideal for use in normal daylight conditions.
- Dark Mode: Displays light text on a dark background to reduce eye strain in low-light conditions.
- System Mode: Aligns the appearance with the operating system's setting, providing a consistent look and feel across different applications.
Here is how to Specify Appearance Mode in Snowflake Snowsight:
Step 1—Sign in to Snowflake Snowsight
Navigate to the Snowflake Snowsight login page and enter your credentials to sign in.
Step 2—Open the User Menu
Once logged in, click on your username at the bottom left right corner to open the user menu.
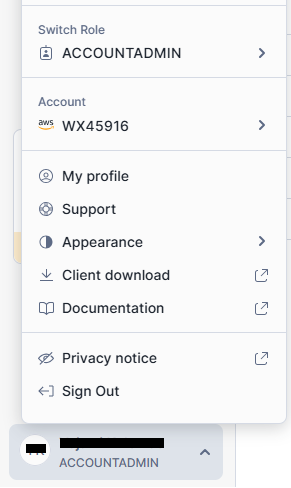
Step 3—Select Appearance Mode
In the user menu, click on Appearance and then choose your preferred appearance mode:
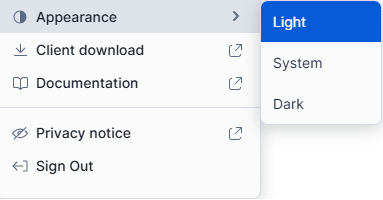
2) Snowflake Notebooks – in public preview
Snowflake Notebooks is a development interface within Snowflake Snowsight, providing an interactive, cell-based programming environment for Python and SQL. It supports exploratory data analysis, machine learning model development, and other data science and data engineering tasks within a single platform. Snowflake Notebooks is now available to all accounts as a preview feature.
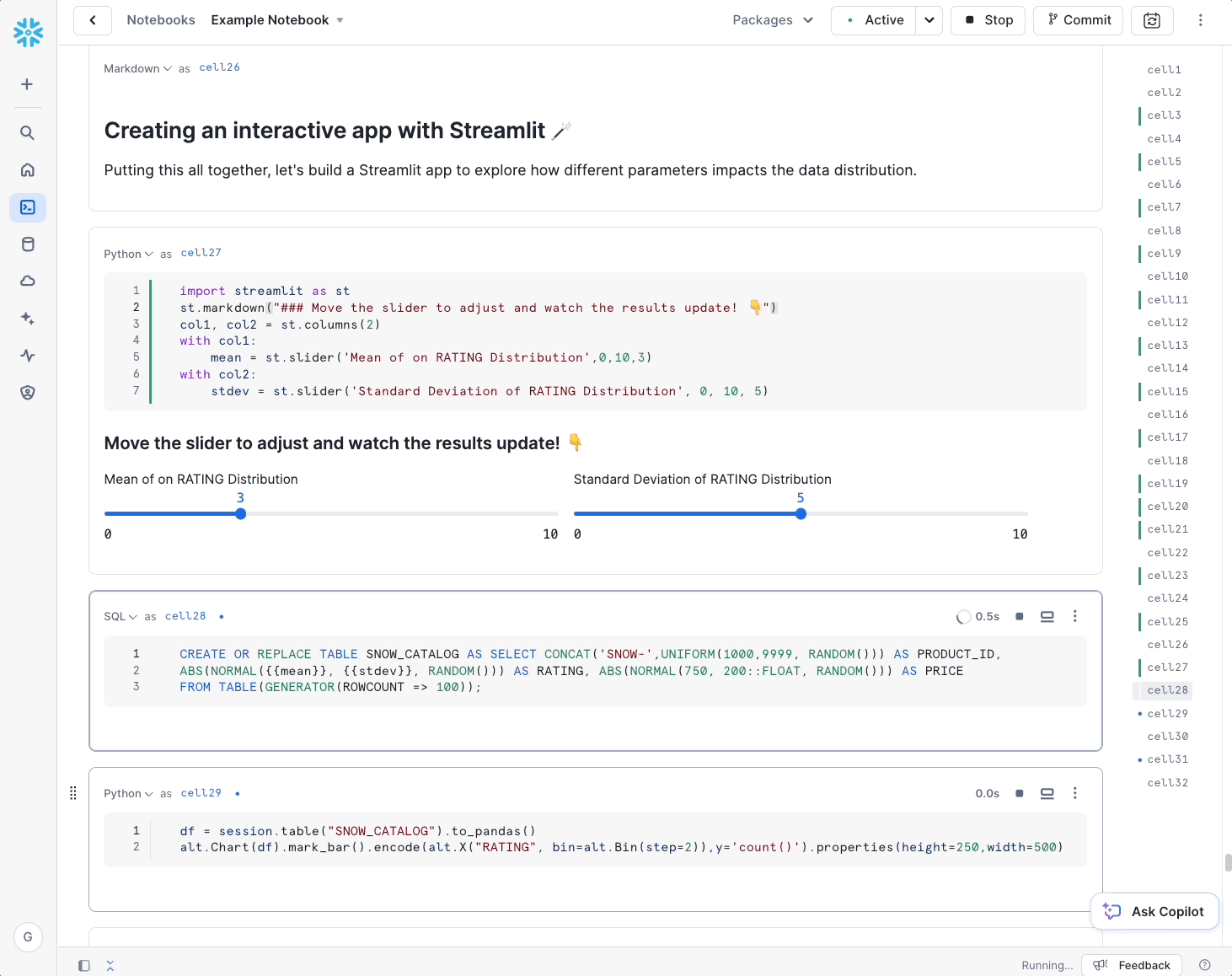
With Snowflake Notebooks, you can now:
- Work with data already in Snowflake or upload new data from local files, external cloud storage, or datasets from the Snowflake Marketplace.
- Write and execute SQL or Python code in a cell-by-cell manner, allowing for quick comparison of results.
- Use embedded Streamlit visualizations and libraries such as Altair, Matplotlib, or seaborn for interactive data visualizations.
- Use Markdown cells to contextualize results and make notes about different outcomes.
- Check data freshness by running a cell along with all preceding modified cells, or debug by executing the notebook cell-by-cell.
- Schedule notebooks to run automatically.
- Use role-based access control and other data governance features to allow collaborative viewing and editing by users with the same role.
Check out this video if you want to quickly get started with your first Snowflake Notebook project.
3) Snowpark Pandas API – in public preview
Snowflake Pandas API, also known as the Snowpark Pandas API, is a feature currently released in preview that allows Python developers to run their pandas code directly on data stored in Snowflake. This API bridges the convenience and familiarity of the pandas library with the scalability, security, and performance benefits of Snowflake's data processing capabilities. Using this API, users can handle considerably larger datasets without having to rewrite existing pandas pipelines for other big data frameworks or employ more expensive hardware.
Here are some fruitful benefits of using Snowflake Pandas API:
- Snowflake Pandas API provides a pandas-compatible layer, making it easy for developers to transition their existing pandas code to Snowflake with minimal changes.
- Snowpark Pandas runs workloads natively in Snowflake, taking advantage of Snowflake's parallelization and query optimization techniques.
- Data remains within Snowflake's secure platform, guaranteeing consistent data access policies and easier auditing.
- Users do not need to manage or tune any additional compute infrastructure, as the API leverages Snowflake's existing engine.
4) Universal Search – Generally available in select regions
As we have earlier mentioned in this article, Snowflake Universal Search helps you to quickly and securely find a wider range of objects than before. Using the Search tab, you can locate tables, functions, databases, data products available in the Snowflake Marketplace, relevant Snowflake Documentation topics, and related articles in the Snowflake Community Knowledge Base.
Snowflake has recently made their Universal Search generally available (GA). Now, with its general availability, Universal Search also includes worksheets and dashboards in your search results. Whether you enter a single word or a complete question in natural language, Universal Search can interpret your query by leveraging your customizable Snowflake asset metadata.
For a detailed guide on Snowflake Universal Search, refer to this article: Snowflake Universal Search 101—Quickly Locate Your Data.
5) Iceberg Tables – Generally available
Snowflake also shared that it is making Iceberg Tables Generally Available, unlocking full storage interoperability for enterprises. Iceberg Tables work like Snowflake native tables but store the table metadata in Apache Iceberg format in the customer-supplied storage. This takes Snowflake’s ease of use, performance, governance, and collaboration to their Iceberg data stored externally.
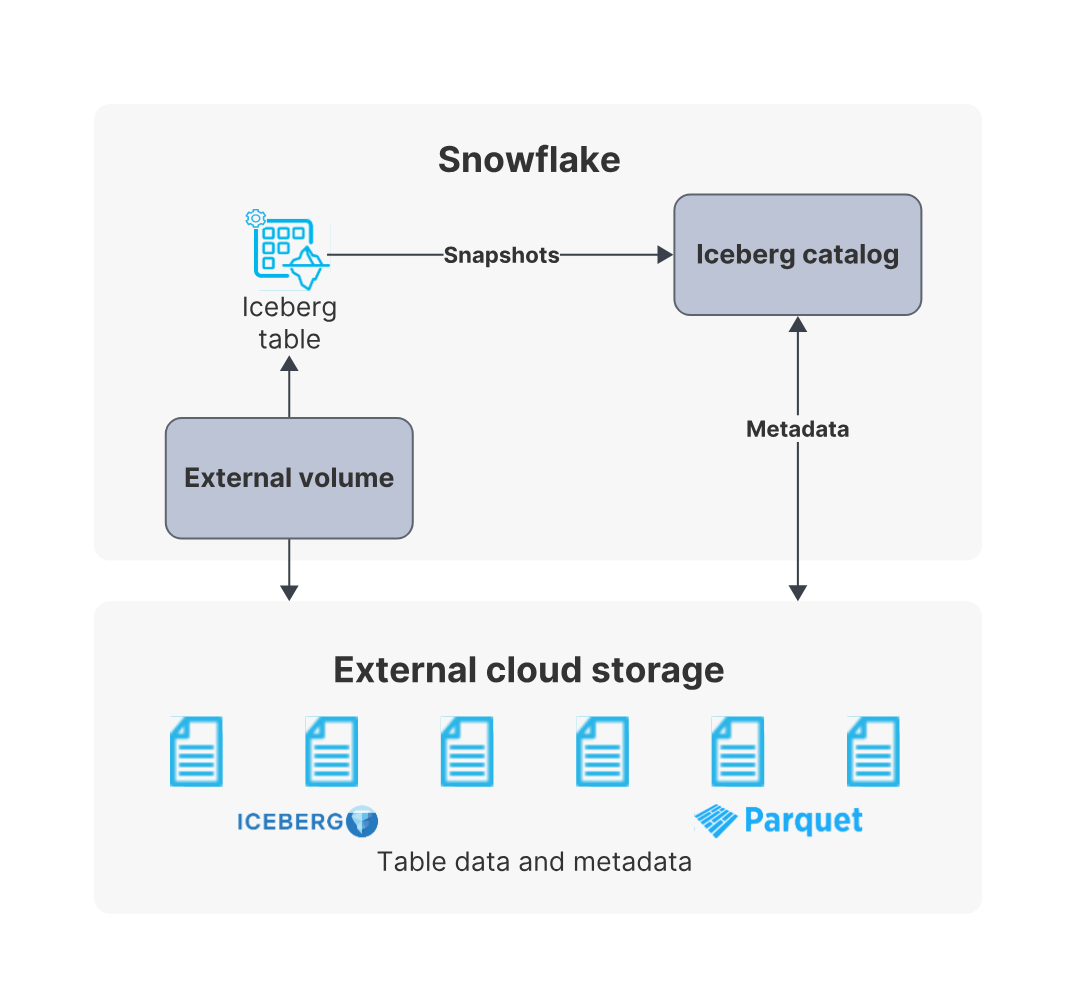
For more in-depth guide on How to use Apache Iceberg with Snowflake, Check out this video:
6) Enhancements to Snowflake Cortex AI
Snowflake also announced major upgrades to its Cortex AI, a fully managed service for building large language model (LLM) applications. The enhancements include a new Snowflake AI & ML Studio—a no-code interface designed to streamline the testing and evaluation stages of the application development workflow.
Alongside this, Cortex AI introduces new Analyst and Search offerings. The Analyst offering facilitates the development of LLM chatbots that allow users to query business data, while the Search offering supports retrieval augmented generation (RAG) and enterprise search applications, utilizing documents and other text-based datasets through enterprise-grade hybrid search.
Not only that, Snowflake also introduced Cortex Guard, a safety mechanism ensuring that chatbots do not generate harmful content in their responses. Also, new MLOps capabilities have been integrated into Snowflake ML, enhancing the overall management and deployment of machine learning models.
Overview of Upgrades and New Features in Cortex AI
1) Snowflake AI & ML Studio (No-Code Interface)
Snowflake AI & ML Studio is a no-code interface designed to simplify the process of testing and evaluating LLM applications. This tool enables users to quickly prototype and validate their LLM applications without the need for extensive coding, making it more accessible to a broader range of users.
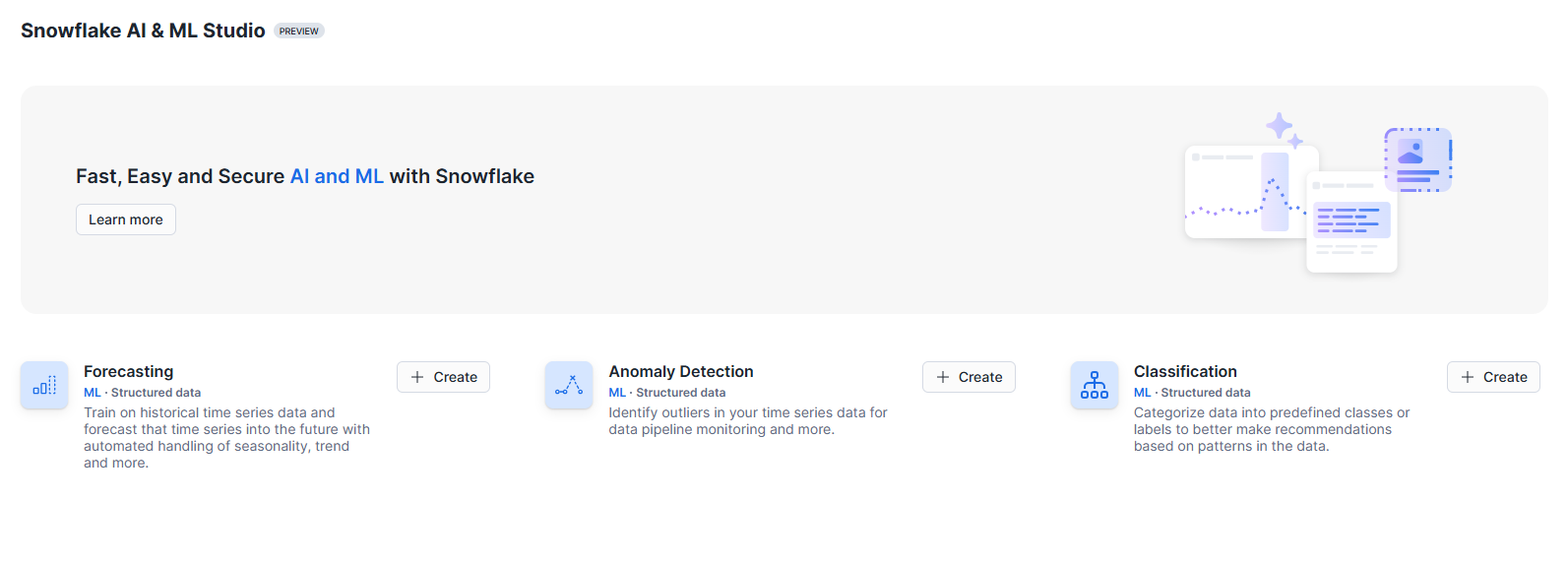
2) Analyst Offering
The Analyst offering in Cortex AI focuses on developing LLM chatbots that help users query and interact with their business data. This feature leverages natural language processing to enable more intuitive and effective data analysis, allowing users to extract insights and information from their datasets through conversational interfaces.
3) Search Offering
The Search offering supports retrieval augmented generation (RAG) and enterprise search applications. This functionality enables the integration of documents and other text-based datasets into search applications, providing powerful and precise enterprise-grade hybrid search capabilities. This is particularly useful for applications requiring comprehensive and accurate information retrieval from large volumes of text data.
Additional Enhancements
1) Cortex Guard
Cortex Guard is a safety mechanism designed to ensure that chatbots created using Cortex AI do not produce harmful or inappropriate content. This feature is crucial for maintaining the integrity and trustworthiness of AI-generated responses, particularly in sensitive or business-critical applications.
2) New MLOps Capabilities
The new MLOps capabilities in Snowflake ML enhance the management, deployment, and monitoring of machine learning models. These capabilities provide tools and frameworks for automating various aspects of the ML lifecycle, including model training, versioning, and deployment, thereby improving the efficiency and reliability of machine learning operations.
7) Document AI – in public preview
Snowflake also made Document AI available in preview, accessible to accounts in AWS and Microsoft Azure commercial regions. Document AI uses Arctic-TILT, Snowflake’s proprietary large language model (LLM), to extract data from various document formats, including text-heavy paragraphs and graphical content like logos and handwritten text. Document AI supports both zero-shot extraction and fine-tuning, allowing for continuous processing of specific document types, such as invoices or financial statements.
For a detailed explanation of how Snowflake Document AI works and its components, refer to this article: HOW TO: Extract Data From Documents via Snowflake Document AI.
8) Polaris Catalog
Snowflake has introduced the Polaris Catalog, a vendor-neutral, open catalog implementation designed for Apache Iceberg. This catalog is designed to enhance interoperability and control over enterprise data, allowing organizations to manage their data with greater flexibility and security.
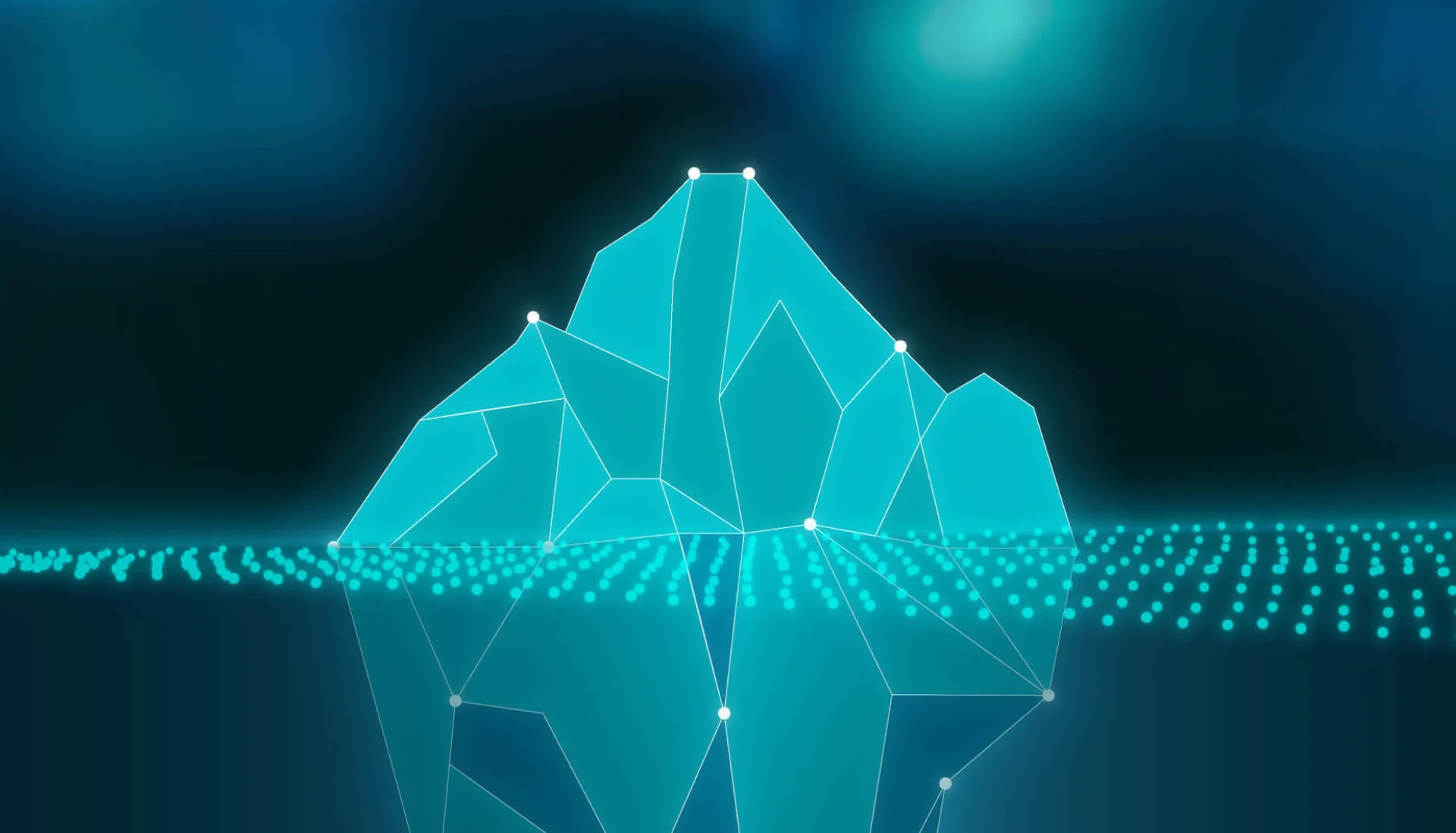
The Polaris Catalog is built on the standards set by the Apache Iceberg community. It uses Iceberg’s open REST API protocol to provide a centralized place where any engine can find and access an organization’s Iceberg tables. This catalog supports multiple processing engines, including Apache Flink, Apache Spark, Dremio, PyIceberg, Trino and Snowflake iteslef, which enables enterprises to use a single copy of data across various tools without vendor lock-in.
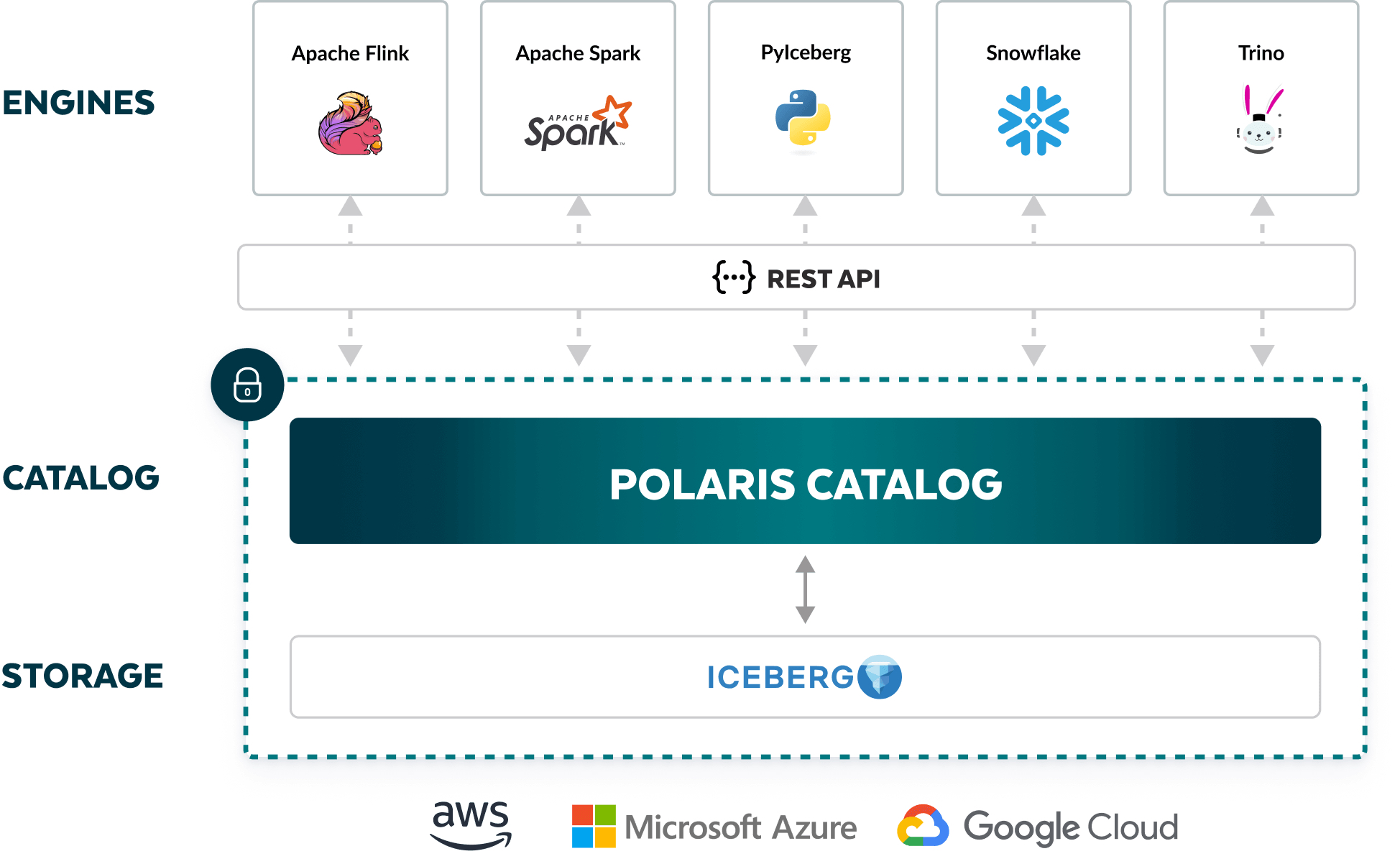
Here are some of the key features of Snowflake Polaris Catalog:
1) Cross-Engine Interoperability—Snowflake Polaris Catalog enables seamless read and write operations across different engines, ensuring that data architects can use multiple engines concurrently without needing to move or copy data. This reduces storage and compute costs and enhances data processing efficiency.
2) Open Source and Flexible Deployment—Snowflake Polaris Catalog can be hosted on Snowflake’s AI Data Cloud or self-hosted using containers such as Docker or Kubernetes. This flexibility allows organizations to choose their preferred hosting infrastructure, further eliminating vendor lock-in.
3) Enterprise Security—Snowflake Polaris Catalog maintains consistent security and governance policies, integrating with Snowflake Horizon’s governance features such as column masking, row access policies, object tagging, and sharing.
For more in-depth guide on Snowflake Polaris Catalog, Check out this video:
9) Snowflake Horizon Marketplace
Snowflake launched Horizon to provide organizations with a comprehensive set of governance features—compliance, security, privacy, interoperability, and access—to manage and govern data, applications, and models effectively across their ecosystems.
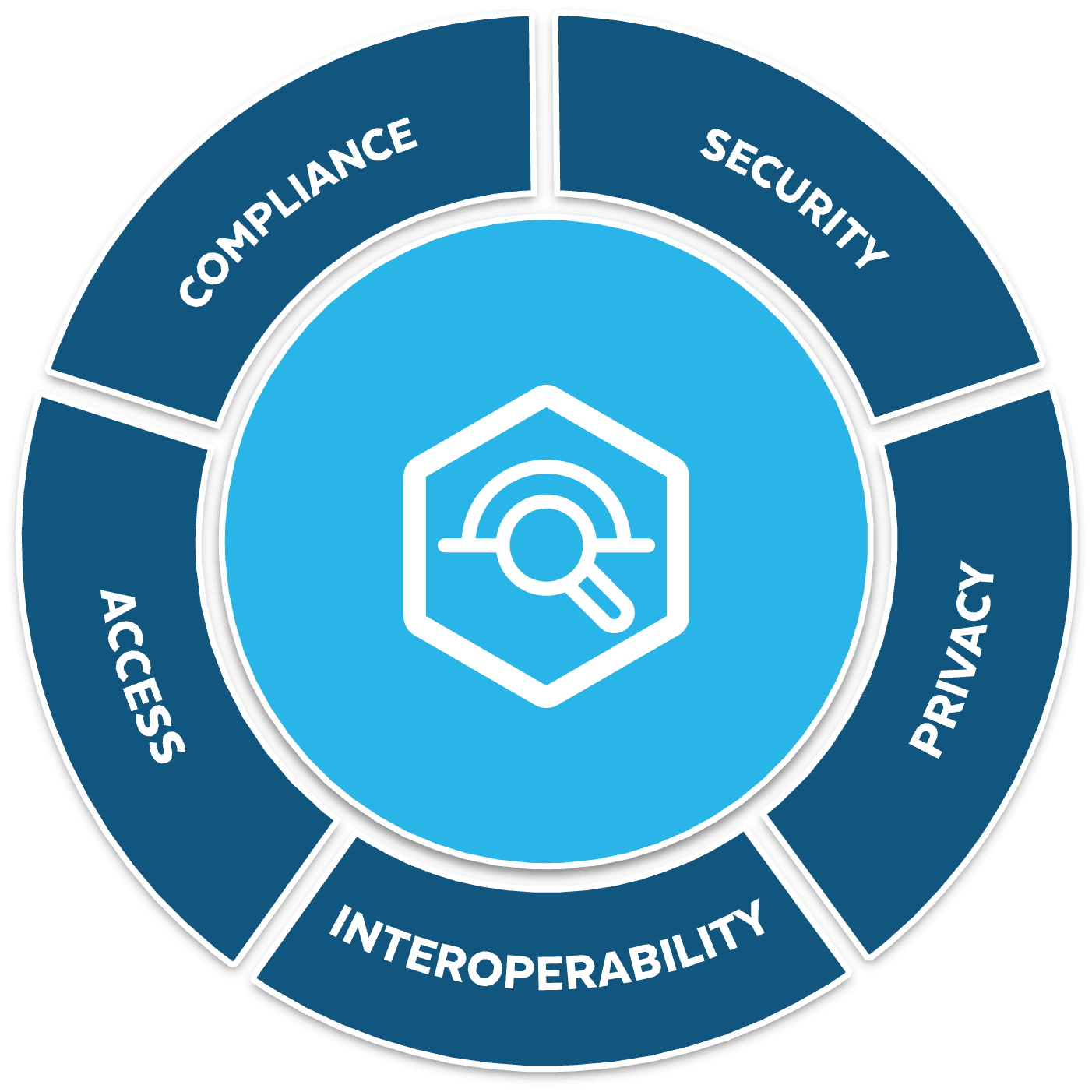
Snowflake Horizon is a built-in governance solution that integrates seamlessly with the Snowflake platform. It offers a unified platform for managing data compliance, security, privacy, interoperability, and access, making it easier for organizations to govern their data assets both inside and outside their environments.
Here are some of the core capabilities of Snowflake Horizon:
1) Compliance—Horizon includes audit histories, data quality monitoring, and data lineage tracking, guaranteeing robust compliance with various regulatory requirements.
2) Security—The platform provides continuous risk monitoring, role-based access control (RBAC), and granular authorization policies. The new Trust Center offers centralized security monitoring and compliance with industry best practices, reducing total cost of ownership and enhancing security.
2) Privacy—Snowflake Horizon supports advanced privacy features such as dynamic data masking, aggregation, projection policies, and differential privacy policies.
3) Interoperability—Snowflake Horizon facilitates integrations with other Apache Iceberg-compatible catalogs and engines, as well as leading data catalog and governance solutions.
4) Access—The platform enhances data discovery and sharing capabilities through features like object tagging, direct data and app sharing, and a new internal marketplace for curating and publishing data products within the organization.
10) Snowflake Trail (Observability)
Finally, Snowflake announced something big called Snowflake Trail—a suite of capabilities designed for developers to better monitor, troubleshoot, debug, and take action on pipelines, applications, user code, and compute utilizations.
Snowflake Trail is a set of observability features that provide developers with comprehensive insights into the performance of their Snowflake environments. It simplifies the process of telemetry, making it easier to gain visibility into application and pipeline performance without the need for complex setups or additional data transfers.
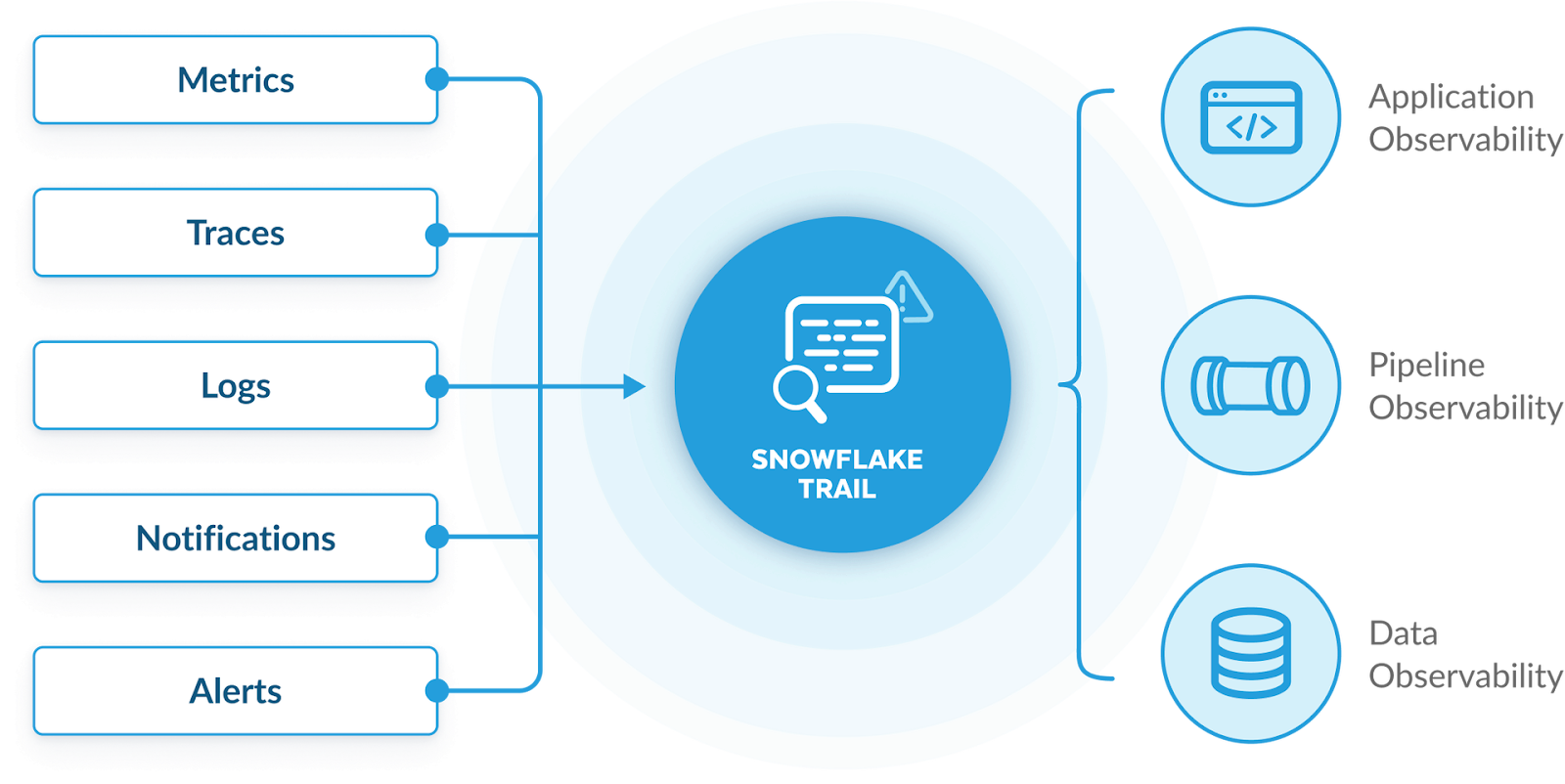
Here are some Key features of Snowflake Trail:
1) Effortless Telemetry—Snowflake Trail eliminates the need for any kind of third party agent installation, time-consuming setups, and data export tasks. With a single setting, users can easily get immediate insights into the performance and resource usage of their Snowpark code, enabling quick diagnosis and debugging of applications and pipeline developments.
2) Super Fast Insights—Events are processed within Snowflake, providing real-time performance data without the need for external data transfers.
2) Reduce Time to Detect (TTD) and Time to Resolution (TTR)—Snowflake Trail offers a comprehensive set of telemetry signals, including metrics, logs, and span events—all integrated within Snowflake Snowsight.
3) Bring Your Own Tools (BYOT) or Use Snowsight—Snowflake Trail's smooth interaction with well-known developer tools like Datadog, Grafana, Metaplane, Monte Carlo, PagerDuty, and Slack is made possible by its OpenTelemetry standards-based architecture. Also, developers can use Snowsight to monitor and trace their pipelines, applications, and runtime usage directly within Snowflake.
Save up to 30% on your Snowflake spend in a few minutes!
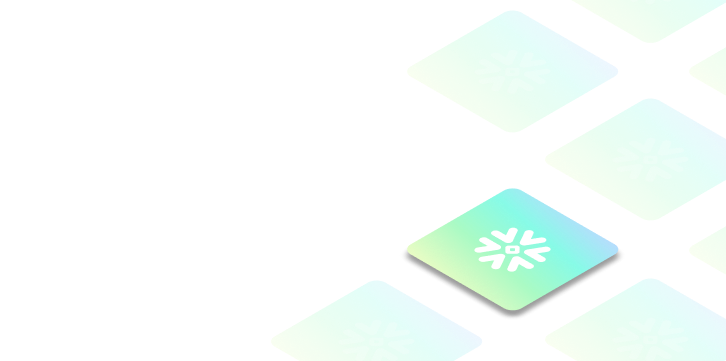
There you go, that's all Snowflake has announced and released at the Snowflake Data Cloud Summit 2024.
Conclusion
And that's a wrap! Wow, what a year it's been for Snowflake! Their 2024 updates and releases have totally shaken up the world of data-driven decision-making and AI. Snowflake's dedication to making AI accessible within the data cloud has never been more evident, with significant advancements designed to boost efficiency, scalability, and innovation for enterprises. We're stoked to see what other brilliant ideas they come up with next.
In this article, we have covered:
- Core Snowflake AI Updates and Releases in 2024
- More Enhanced Snowflake AI Capabilities—and Integrations
- Latest Collaborations and Partnerships
- Snowflake Data Cloud Summit 2024 — Announcements and Releases
… and so much more!
FAQs
What is Snowflake Arctic?
Snowflake Arctic is a state-of-the-art large language model developed by Snowflake's AI research team, featuring a Dense Mixture of Experts (MoE) Hybrid transformer architecture with 480B parameters distributed among 128 fine-grained experts, and top-2 gating to choose 17B active parameters.’
What is Snowflake Cortex?
Snowflake Cortex is a fully managed service that integrates advanced AI and ML solutions directly within the Snowflake environment, enabling users to leverage their data for analysis, building AI applications, and utilizing pre-built models and vector search capabilities.
What is Snowflake Universal Search?
Snowflake Universal Search is a powerful search functionality that allows users to quickly find a wide array of objects and resources across their accounts, including database objects, data products in the Snowflake Marketplace, relevant documentation, and community knowledge base articles using natural language queries.
What is Snowflake Copilot?
Snowflake Copilot is an LLM-powered assistant designed to simplify data analysis within the Snowflake ecosystem, assisting users in data exploration, SQL query generation, and understanding Snowflake features through natural language requests.
What is Snowflake Document AI?
Snowflake Document AI uses a proprietary large language model called Arctic-TILT to extract data from various types of documents, including text-heavy content and graphical elements like logos, images, and handwritten text.
What is the VECTOR data type in Snowflake?
The VECTOR data type in Snowflake is designed to efficiently encode and process vector data, supporting applications that require semantic vector search and retrieval.
What are Snowflake Data Clean Rooms?
Snowflake Data Clean Rooms provide a secure and privacy-preserving environment where multiple parties can collaborate and analyze data without revealing their raw data, using techniques such as differential privacy and encrypted multi-party computations.
What are Snowflake Native Apps?
Snowflake Native Apps allow developers to create and distribute data applications within the Snowflake ecosystem, leveraging Snowflake's core functionality and enabling seamless data sharing and business logic execution across different Snowflake accounts.
What is Snowflake Horizon?
Snowflake Horizon is a built-in governance solution that provides organizations with a comprehensive set of governance features—compliance, security, privacy, interoperability, and access—to manage and govern data, applications, and models effectively across their ecosystems.
What is Snowflake Trail?
Snowflake Trail is a suite of observability features that provide developers with comprehensive insights into the performance of their Snowflake environments, simplifying the process of telemetry and making it easier to gain visibility into application and pipeline performance without the need for complex setups or additional data transfers.